Rating the robots: Artificial intelligence for literature reviews
By Malia Gill, MS, Kimberly Ruiz, EdM, Fi Stewart, MSc, Erika Wissinger, PhD
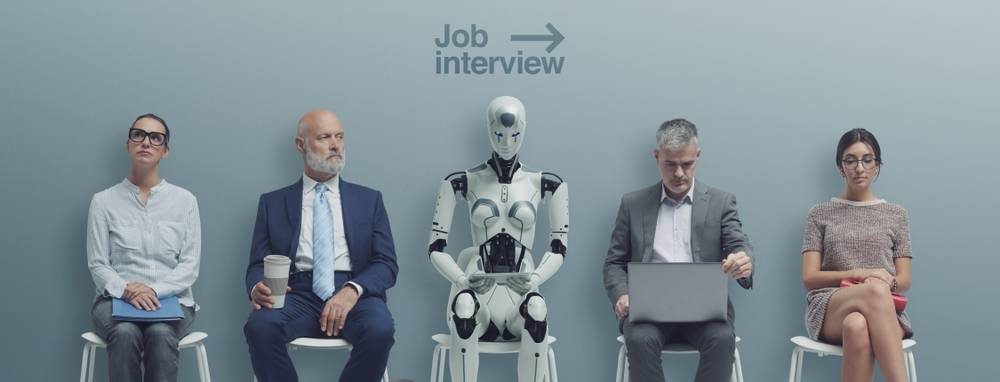
The need for evidence synthesis innovation
Literature reviews are the primary method of identifying and synthesizing available scientific evidence on a given topic. The most comprehensive type of review, a systematic literature review (SLR), is a valuable tool that answers key research questions (KRQs) by identifying all relevant evidence using rigorous and reproducible methods. SLRs are considered the “gold standard” in evidence-based medicine (EBM), having long been placed at the top of the EBM hierarchy, and they are used by a wide range of healthcare professionals and industry stakeholders. SLRs are also essential for informing practice and policy decisions and are required by many regulatory and health technology assessment agencies for submissions. Targeted literature reviews (reviews with less stringent methods) also play an important role in assessing treatment and disease landscapes and guiding strategy in early-stage drug development.
Although literature reviews are essential tools for EBM decision-making, they are both time- and labor-intensive. One analysis found that the mean time to complete and publish an SLR was 67 weeks, and pharmaceutical companies spend millions of dollars every year conducting literature reviews. In addition to time and cost barriers, literature reviews may already be out of date upon completion due to the rapidly increasing number of published articles and available journals. Other potential limitations of literature reviews include possible bias and lack of transparency inadvertently introduced by human reviewers.
Artificial intelligence (AI) is a promising technology to address some of these limitations by improving the efficiency of literature reviews and reducing time and workload burden. Many companies, including Cencora, now offer literature reviews that incorporate AI-assisted processes. While the potential benefits of AI are exciting, it is crucial that AI-assisted processes are validated to maintain methodological rigor and accuracy. To gain greater insight into the performance of AI for specific phases of the literature review process, we assessed the functionality and capabilities of 4 AI platforms for publication screening and 3 AI platforms for data extraction. We chose to assess these platforms because they were referenced in published scientific literature, widely available for public use, or advertised to have novel AI capabilities. Here we provide the findings from our assessment and a general overview of using AI for literature reviews.
Identifying relevant literature
A typical literature review requires multiple reviewers to examine hundreds, sometimes thousands, of potentially relevant publications, beginning with reading the title and abstract of each publication (title/abstract [TIAB] screening) to determine relevance for the KRQs. One possible application of AI is to identify relevant studies from thousands of references retrieved by comprehensive literature searches at a speed considerably faster than humans.
Reference screening using an AI training set
Among the AI platforms we assessed, the most common AI-assisted method of literature screening was training an AI model with a subset of publications screened by human reviewers. Following the training, the model could then be used to predict the relevance of the remaining publications at the TIAB screening level.
When we compared AI-assisted TIAB screening on the same set of references across multiple platforms, the results were mixed. In terms of successfully including relevant publications, some AI models performed far worse than humans (sensitivity, i.e. true positive rate, <40%) while other AI models achieved similar results to their human counterparts (sensitivity >90%). However, AI models that were able to successfully identify the majority of relevant publications (high sensitivity) were generally worse at excluding non-relevant publications (low specificity, i.e. true negative rate) as demonstrated with Platform 2 (Table 1).
AI-assisted TIAB screening can be used as a first pass to exclude clearly irrelevant publications, with the remaining publications screened by humans at the TIAB and full-text levels. When utilizing this process, AI-assisted screening using platforms with high sensitivity (e.g. Platform 2) can reduce the time needed for TIAB screening by nearly 50%.
Training an AI model using a subset of references allows AI-assisted screening to be customized for each new project, but this method does have limitations. If a particular outcome, such as healthcare resource use, is not reported in any of the training set references, then it will not be recognized by the AI model as a relevant outcome. Therefore, AI-assisted screening is better suited to disease areas with a large volume of published literature on the full range of outcomes of interest. Even with a robust training set, there is still a possibility of missing relevant articles. Although the best AI models included the majority of relevant publications (high sensitivity), SLRs adhere to rigorous methods where no relevant articles should be excluded. There is some risk of missing relevant articles with AI-assisted screening. When used with SLRs, a third reviewer would likely identify relevant articles when reviewing the AI vs human reviewer conflicts. When AI is used with non-systematic reviews, human quality checks of excluded references are recommended to ensure accuracy. Finally, we only evaluated the training set method of AI-assisted screening for screening at the TIAB level. Although some AI platforms may be able to screen full-text publications, there are considerable difficulties with variation in publication file format and with interpreting tables and figures.
Reference screening using large language models
Another method of AI-assisted literature screening is to interrogate potentially relevant sources using large language models (LLMs). In this method, the literature review eligibility criteria (eg, population, interventions, comparators, outcomes, study design) are translated into yes/no questions. Then, the LLM is asked to assess the relevance of references retrieved by literature database searches based on the answers to the eligibility questions. While this method does not require a training set for each new project, the accuracy heavily depends on the phrasing of the eligibility questions. Even when the eligibility questions were carefully revised, this AI-assisted method was worse than human reviewers at including relevant references (sensitivity <40%; Table 1). For example, when the LLM from Platform 3 was asked whether studies reported on “healthcare resource use such as healthcare practitioner visits, inpatient events, hospital length of stay, emergency department visits, intensive care unit events, and intensive care unit length of stay,” studies reporting hospitalizations were excluded. In this case, hospitalizations were not recognized as a healthcare resource use outcome since hospitalizations were not explicitly mentioned in the eligibility question. In similar situations, human reviewers can make thoughtful connections and inferences to ensure that all relevant outcomes are included. Most LLMs were developed for general purposes and were not trained extensively on medical and clinical publications and documents. This may make LLMs less successful at interpreting highly specialized scientific terminology. In contrast, experienced literature reviewers and medical professionals with expertise in their specific therapeutic area can create better prompts and frameworks to answer research questions. This emphasizes the importance of human involvement even when using AI-assisted methods.
Based on our assessment of AI-assisted screening, some AI tools can be utilized to accelerate review timelines while still identifying the relevant scientific evidence, particularly for topics with a large volume of published literature on clearly defined outcomes of interest. However, human reviewer guidance and oversight are still necessary to ensure that relevant evidence is not missed.
Table 1. AI-assisted screening for an SLR
SLR (total references = 2,613)a | Platform 1 | Platform 2 | Platform 3 |
---|---|---|---|
Description of AI-assisted screening | ~20% of references used to train AI model | ~20% of references used to train AI model |
LLM interrogated using SLR eligibility questions |
Sensitivity | 32%-37%b |
93% |
38% |
Specificity | 99% |
51% | 95% |
# of publications included in the final SLR excluded by the AI during TIAB screening |
Data not captured |
0 (0%) |
17 (33%) |
Key: AI – artificial intelligence; LLM – large language model; SLR – systematic literature review; TIAB – title/abstract.
a The fourth platform was not assessed on the same set of data. Therefore, the results are not included in this table.
b Range is reported because multiple training sets were assessed for Platform 1.
Extracting relevant data
AI tools and platforms have been developed to assist with the time-consuming data extraction component of the literature review process. We assessed an AI-assisted data extraction tool that functioned in a similar way to the LLM interrogation method of AI-assisted screening. Outcomes of interest were phrased as questions (e.g. what is the mean age of the study population?), and AI identified the location in the article where the answer could be found. At this point, the human reviewer would extract the relevant data. A large benefit of AI-assisted data extraction is data provenance—all extracted information is linked to the exact location where it is reported in the full-text article.
Our assessment of AI-assisted data extraction did not result in any meaningful time savings compared to extraction completed by human reviewers. Furthermore, the AI tool we assessed for data extraction had many limitations. The outcomes of interest were not always accurately identified in the article. The identification process was also limited if the outcomes of interest were discussed in more than one place in the text, or if there were synonyms for the outcome description (e.g. deaths vs mortality). Moreover, data reporting in scientific publications is not standardized, and data can be easily missed by AI. The AI tool we assessed was not able to interpret tables or figures, which are important sources of reported data. These are not impediments to experienced human reviewers who can recognize potentially related terms and synthesize data reported in different parts of a publication (e.g. methods, results, figures/tables, discussion).
While AI-assisted data extraction remains an area of interest, the benefits of current methods are limited based on our assessment. However, there are other AI tools and platforms that promise additional AI-assisted data extraction capabilities. Tools that extract data in numerical/text form, in addition to signaling the location of data and multimodal tools that can interpret graphs and images, are becoming available to users. As AI-assisted data extraction improves, it will remain important to validate new processes.
The state of AI for literature reviews
AI is a promising and powerful technology, and it has the potential to make a significant impact on the world of research and evidence synthesis. While AI can be used to increase the efficiency of literature reviews, it has some limitations. AI cannot accurately conduct an entire literature review without considerable input from researchers and medical professionals, and accuracy is of the utmost importance when researching topics that could impact people’s health and healthcare. AI functions like a new, untrained researcher. Experienced professionals are needed to guide and validate AI results.
Current best practices emphasize transparency. The 2020 PRISMA guidelines allow the use of automation tools, such as AI, but specify that the details about their use, training, and validation should be described. As AI continues to develop and improve in the literature review space, researchers must be vigilant that tools have been appropriately validated and must ensure transparency around their use.
Ultimately, validated AI tools in the hands of experienced researchers have the potential to streamline the literature review process and efficiently provide important research insights. While the precise role of AI in literature and evidence synthesis is evolving, there is undoubtedly scope for AI to mature into an essential tool for literature reviewers, provided there is a solid basis of training and validation in its development.
- Borah R, Brown AW, Capers PL, Kaiser KA. Analysis of the time and workers needed to conduct systematic reviews of medical interventions using data from the PROSPERO registry. BMJ Open. 2017 Feb 27;7(2):e012545. doi:10.1136/bmjopen-2016-012545
- Michelson M, Reuter K. The significant cost of systematic reviews and meta-analyses: a call for greater involvement of machine learning to assess the promise of clinical trials [published correction appears in Contemp Clin Trials Commun. 2019 Sep 12;16:100450]. Contemp Clin Trials Commun. 2019 Aug 25;16:100443. doi:10.1016/j.conctc.2019.100443
- OCEBM Levels of Evidence Working Group. The Oxford 2011 Levels of Evidence. Oxford Centre for Evidence-Based Medicine. https://www.cebm.ox.ac.uk/resources/levels-of-evidence/ocebm-levels-of-evidence
- Open AI. What is ChatGPT? Accessed 31 January 2024. https://help.openai.com/en/articles/6783457-what-is-chatgpt
- Page M J, McKenzie J E, Bossuyt PM, et al. The PRISMA 2020 statement: an updated guideline for reporting systematic reviews. BMJ. 2021;372:n71 doi:10.1136/bmj.n71
- Wallace SS, Barak G, Truong G, Parker MW. Hierarchy of Evidence Within the Medical Literature. Hosp Pediatr. 2022;12(8):745-750. doi:10.1542/hpeds.2022-006690
Additional insights
Insight
HTA Quarterly Fall 2024
AmerisourceBergen
August 2024
In this special themed edition, our colleagues at Vintura, a Cencora company, explore the potential of value-based partnerships (VBPs). First outlining the principles, goals and potential benefits of VBPs, this edition also provides two case studies that demonstrate how successful partnerships work in practice.