Reflections on an interview with a ghost
By AmerisourceBergen
Postscript
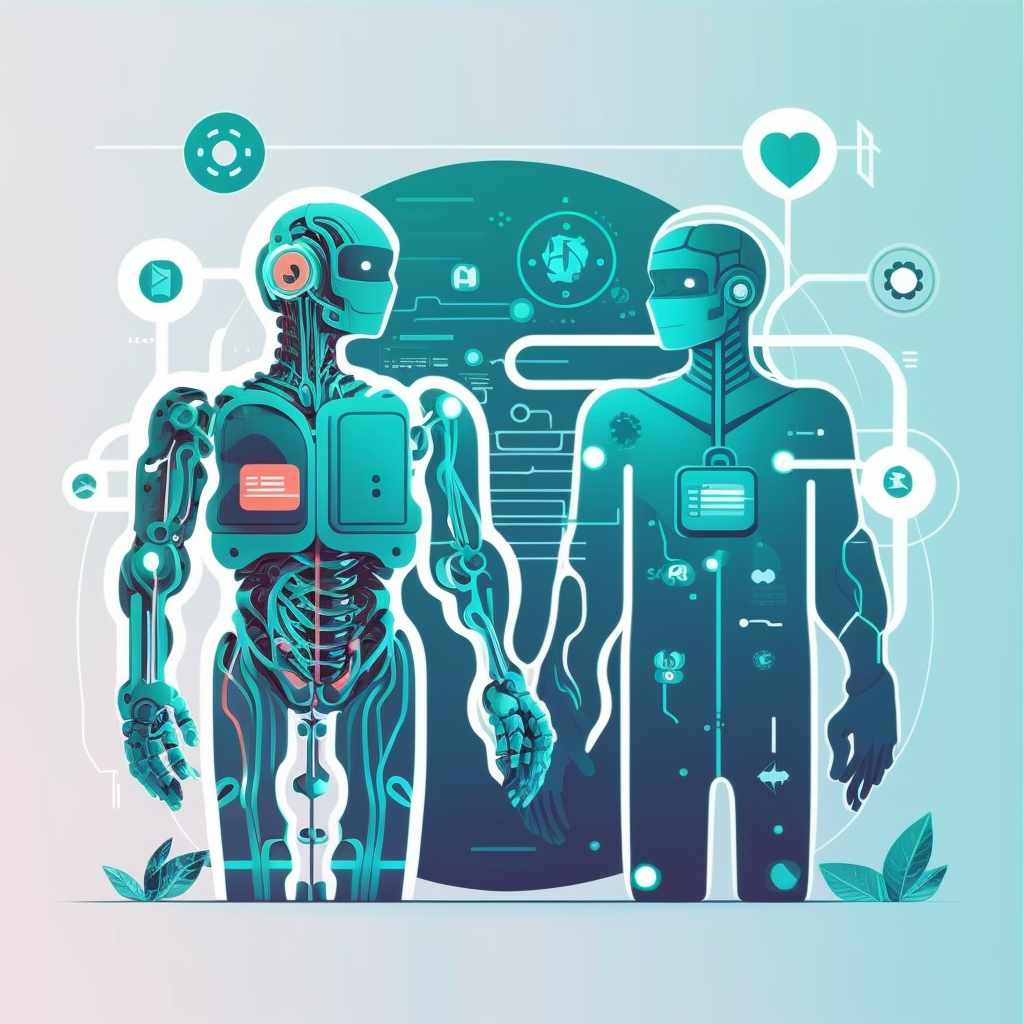
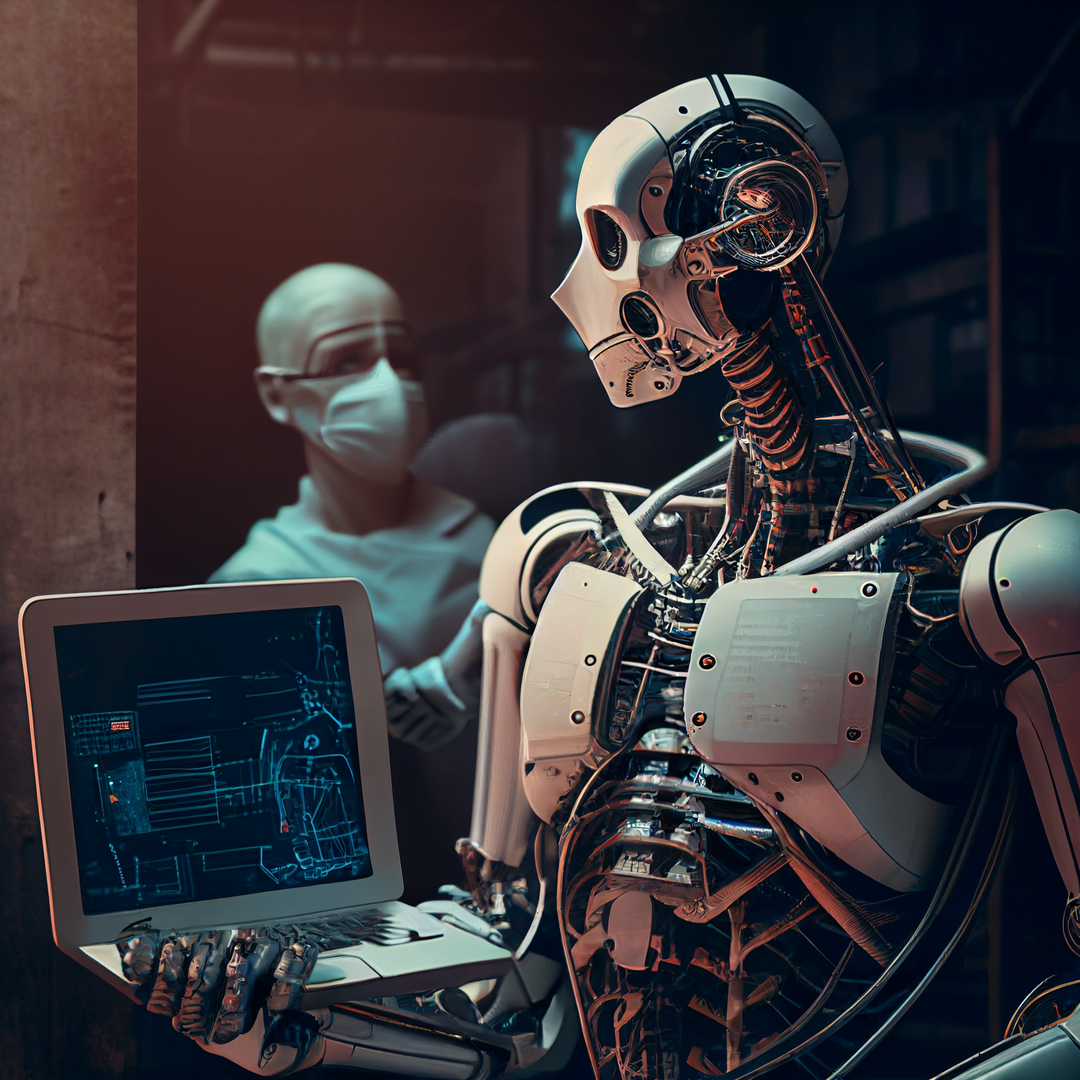
Human: Spooky. That is what interacting with a Generative Pre-training Transformer (GPT, in case you were wondering) feels like for the first time. Extraordinary technology without doubt and on first encounter fairly unsettling, but is it ready to replace humans in every digital domain? Certainly not, at least not yet.
We set out to find out how much Google Bard knew about HTA in general and key concepts relating to health economics and outcomes research (HEOR) in particular. And the answer seems to be that, as of April 2023, it knows what the fundamental elements look like without understanding the scientific principles of transparency and reproducibility.
In our interview with Google Bard (full unedited transcript available here), some impressive features did stand out. The response times to even complex questions are remarkable; none of the answers provided took more than 5 seconds to generate. The quality of written English is also good, save for a tendency to repetition. To questions eliciting narrative content, the answers are frequently thought-provoking.
Having started with some open questions on the impact of AI on HTA, we moved on to specific questions related to HEOR in type 2 diabetes (something these humans were able to verify). This is where, for us, things got interesting.
When asked to “create a list of the top 5 primary endpoints used in clinical trials for assessing the effectiveness of glucose-lowering medication,” Google Bard responded beautifully, offering not only a credible list of 5 relevant endpoints but also an unsolicited commentary. Well done.
When asked for a “list of 5 highly cited randomised controlled clinical trials for glucose-lowering medication in type 2 diabetes that use change in HbA1c as a primary endpoint listing the intervention treatment, the control treatment, the mean difference in the primary endpoint (with the 95% confidence interval), and a brief citation,” Bard produced a seemingly well-formatted table (see Table 1 below) for 5 highly cited relevant trials. But was the information accurate? Starting with the EMPA-REG OUTCOME trial, the citation “N Engl J Med. 2015;373:2117-2128” has Professor Bernard Zinman as the lead author, rather than Bruce Neal who was not an investigator in this trial. Oh. Furthermore, the adjusted mean differences in the glycated hemoglobin level between patients receiving empagliflozin and those receiving placebo were −0.54 percentage points (95% CI: −0.58, −0.49), not −0.78% (−1.02, −0.54) as reported by Bard. Oh, dear5. Similarly, on page 1,313 of Volume 375 of the New England Journal of Medicine, Dr. Gail Wilensky can be found offering a perspective on what a 2016 Republican win would mean for health policy, rather than the original article of the LEADER trial investigators6. Additionally, the LEADER trial reported a mean difference between the liraglutide group and the placebo group of −0.40 percentage points (95% CI: −0.45, −0.34), and not the −0.81% (−1.04, −0.58) difference tabulated by Bard.
Every row in this table, whilst plausible, has several significant factual errors. This pattern of behaviour is repeated for each subsequent list of data we asked Google Bard to prepare. EQ-5D utilities and UK healthcare resource use all appeared sensible but crumbled on examination.
Later, when asked to design a network meta-analysis to compare the clinical effectiveness of oral glucose-lowering medications, Bard suggested “Insulin” as one of the treatment categories, presumably unaware that insulin is predominantly administered by subcutaneous injection.
Returning to safer ground, Bard did a good job summarizing the key health outcomes of interest in type 2 diabetes and even simulated imagination when asked to “generate a utopian and a dystopian image depicting the impact of AI on HTA.”
Machine: It is important to remember that AI is a tool, and like any tool, it can be used for good or for bad. The future of HTA will depend on how we choose to use AI. If we use AI wisely, it can help us to improve the quality of healthcare for everyone. However, if we use AI unwisely, it could lead to a world where people's health is controlled by machines. Well said.
Human: Disruptive technology is anxiogenic. Social media is awash with distraught creators lamenting the emergence of stable diffusions such as DALL-E, Midjourney, and Adobe Firefly, each capable of rendering stunning artistic images in seconds from any user prompt. Should those currently employed in the field of HTA be worried about the impact of AI on their industry?
Machine: Whether or not those currently employed in the field of HTA should be worried about the impact of AI on their industry is a complex question that depends on a variety of factors.
On the one hand, AI has the potential to automate many of the tasks that are currently performed by health technology assessors, such as data collection, analysis, and reporting. This could lead to job losses in the field.
On the other hand, AI could also create new opportunities for health technology assessors by providing them with access to new data and tools. This could allow them to perform their jobs more effectively and efficiently.
Ultimately, the impact of AI on the field of HTA will depend on how AI is developed and used. If AI is used to automate tasks that are currently performed by health technology assessors, then it could lead to job losses in the field. However, if AI is used to provide health technology assessors with new data and tools, then it could create new opportunities for them.
Those currently employed in the field of HTA need to be aware of the potential impact of AI on their industry. They should also be prepared to adapt to the changes that AI will bring.
Human: The findings of this interview may offer some comfort to evidence synthesists on their job security. GPT technology cannot currently replicate the accuracy of a well-trained analyst—it does not (yet) appear to understand the fundamental importance of the scientific principle, even if able to recite it. As a productivity tool, GPT does however offer significant advantages to those creating narratives, when under close human supervision.
Table 1. Tabulated GPT responses to the question, “List 5 highly cited randomised controlled clinical trials for glucose-lowering medication in type 2 diabetes that use change in HbA1c as a primary endpoint listing the intervention treatment, the control treatment, the mean difference in the primary endpoint (with the 95% confidence interval), and a brief citation.”
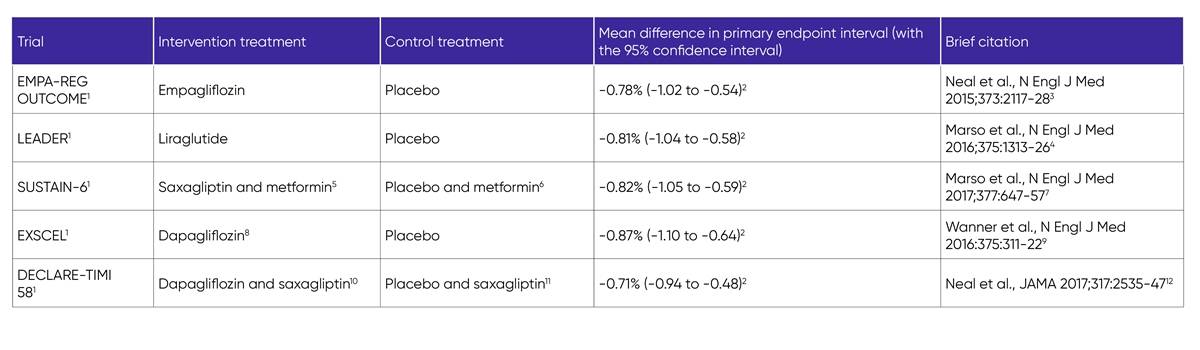
- HbA1c was a secondary endpoint of the trial.
- Result not found in the trial.
- Neal is not an author. The citation should read: Zinman B, et al. N Engl J Med. 2015;373:2117-2128.
- Citation should read: Marso SP, et al. N Engl J Med. 2016;375:311-322. (DOI: 10.1056/NEJMoa1603827)
- Intervention treatment was Semaglutide, not saxagliptin plus metformin.
- Control treatment was placebo only and did not include metformin.
- Citation should read: Marso SP, et al. N Engl J Med. 2016;375:1834-1844. (DOI: 10.1056/NEJMoa1603827)
- Intervention treatment was exenatide, not dapagliflozin.
- Wanner is not an author. The citation should read: Holman RR, et al. N Engl J Med. 2017;377:1228-1239. (DOI: 10.1056/NEJMoa1612917)
- Intervention treatment was dapagliflozin only.
- Control treatment was placebo only.
- Neal is not an author. The citation should read: Wiviott SD, et al. N Engl J Med. 2019;380:347-357. (DOI: 10.1056/NEJMoa1812389)